This blog post provides a brief rundown of the current landscape of autonomous vehicle architecture. It is largely based on an article written by Tom Grey, Director of Product Marketing at Ouster.
To start off, here’s some introductory information for those who might be unfamiliar with autonomous vehicles.
The five-step path to fully autonomous vehicles
The Society of Automotive Engineers has outlined a five-step progression towards fully autonomous vehicles.
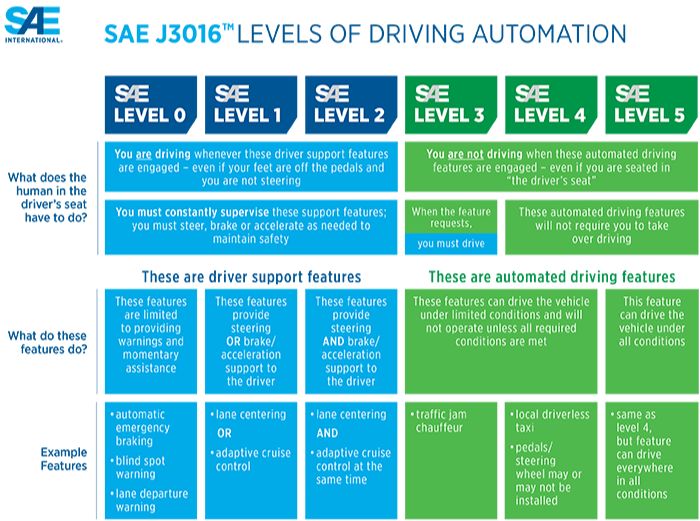
In 2023, commercially deployed vehicles achieve level 2 automation. They rely on a sensor suite of cameras, a front-facing radar, and low-resolution lidar. This hardware runs with a very basic processing stack.
In order to reach SAE level 4 and 5, the industry needs to overcome some major technical challenges:
- Accurate perception and localization in all environments
- Faster real-time decision-making in various conditions
- Reliable and cost-effective systems that can be commercialized in production volumes
Overcoming these problems requires a new hardware stack with a more powerful set of sensors and faster computing infrastructure (that must be cost-effective and scalable).
What types of sensors are usually found in self-driving cars?
1) LiDAR
Level 4 and 5 self-driving prototype cars are all equipped with LiDAR. LiDAR can provide accurate depth and spatial information about the environment. This data, combined with camera and radar data, make the system able to quickly and accurately classify objects around the vehicle.LiDAR data are represented in a 3D point cloud.
Pros:
- LiDAR provide very precise information about depth (range)
- They can be used at any time of day or night, in any kind of weather or lighting
- LiDAR’s mid-level resolution is much better than radar, but lower than a camera
Cons:
- High data rate
- High-quality LiDAR are expensive
- They take up more space (large form factor)
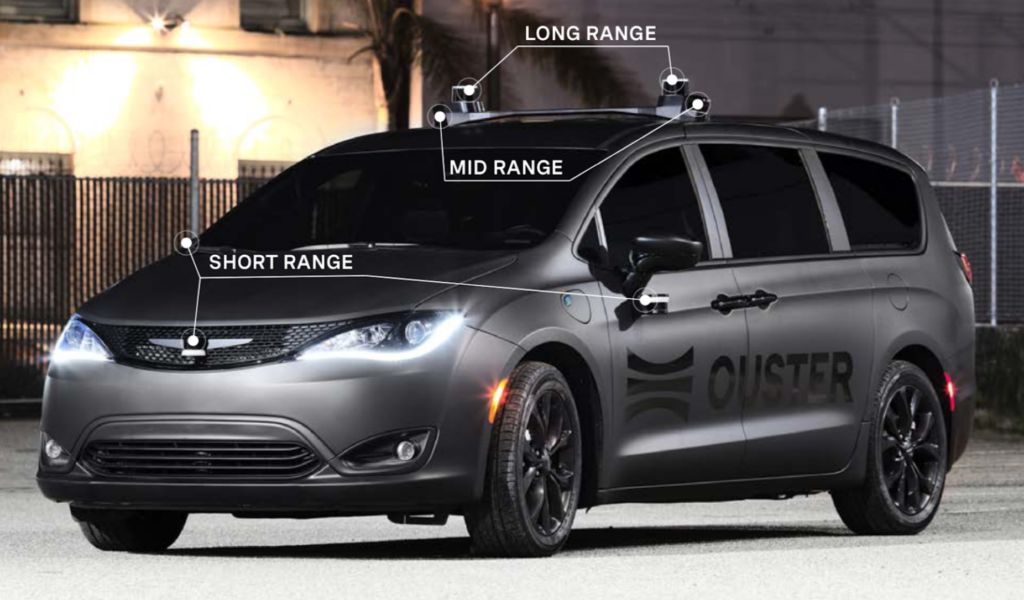
Standard set up of LiDAR sensors (short, mid, long range) on a self-driving car
Integration:
- 4 short-range LiDAR set up around the edges of the vehicle. Their aim is to detect and identify immediately potential risks close to the vehicle (ie. small animals, cones, curbs) that may be even missed by human drivers.
- 2 mid-range LiDAR placed at an angle on the edges of the roof of the vehicle, used for mapping and localization.
- 2 long-range LiDAR set up at the top of the vehicle, used for detecting dark objects and potential obstacles in front of the vehicle when it is traveling at high speeds (360° or forward facing).
2) Camera
Cameras are the traditional core of an autonomous vehicle’s perception stack. Level 4 or 5 vehicles are equipped with over 20 cameras placed around the vehicle, which must be aligned and calibrated to create a very HD 360° view of the surroundings.
Pros:
- High resolution and full color in a 2D array
- Inexpensive
- Easy to integrate, small footprint (can be almost hidden inside the car)
- Sees the world as humans do
Cons:
- Sensitive to changing light conditions and bad weather
- 360° view requires a resource-hungry processing unit to assemble images
3) Radar
Over the past 15 years, radar has become a common sight in automotive applications. Radar provides 3D point clouds of its surroundings through radio waves.
Pros:
- Provides data on the depth of objects and the environment
- Inexpensive
- Robust
- Unaffected by harsh weather conditions (rain, snow)
- Long range
Cons:
- Low resolution
- False negatives with stationary objects and critical obstacles
Integration:
- For Level 4 and 5 autonomous vehicles, the radar is positioned 360º around the vehicle and serves as a reliable and redundant unit for object detection.
- It is recommended to place up to 10 radards around the vehicle.
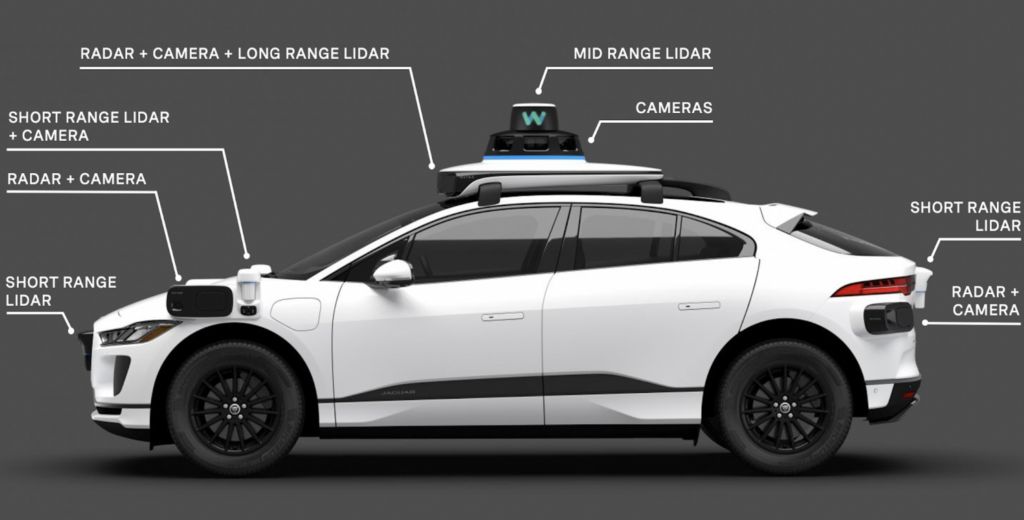
These three sensors each have their pros and cons. However, when combined, they make up a powerful sensor package that enables Level 4 and 5 autonomous driving.
Given the number of sensors deployed on these vehicles, the processing requirements are enormous. Let’s take a look at the different options for meeting this challenge.
Autonomous vehicle processors
There are two main approaches to processing the huge amount of data collected by all these sensors: centralized processing or distributed processing (or Edge Computing).
1) Centralized processing
With centralized processing, all raw sensor data is sent to and processed by a single central processing unit.
Pros: sensors are small, inexpensive and energy-efficient.
Cons: requires expensive chipsets with high processing power and speed. Potentially high application latency, as adding sensors requires additional processing demands.
2) Distributed processing (Edge computing)
With Edge Computing, only the relevant information from each sensor is sent to a central unit, where it is compiled and used for analysis or decision-making.
Pros:
- Lower bandwidth
- Cheaper interface between sensors and CPU
- Lower application latency, less processing power required
- Adding extra sensors won’t significantly increase performance requirements
Cons : higher functional safety requirements
There are CPUs particularly suited to Edge Computing, such as the NVIDIA Jetson Orin.
Edge Computing is fast becoming the dominant choice for autonomous vehicles, as it enables the growing number of data-intensive sensor signals to be processed simultaneously and in real time.
To demonstrate the potential of this solution, the engineers at Ouster used a software called Teraki to merge an Ouster LiDAR with an HD camera.
The Teraki software, an efficient solution for data processing
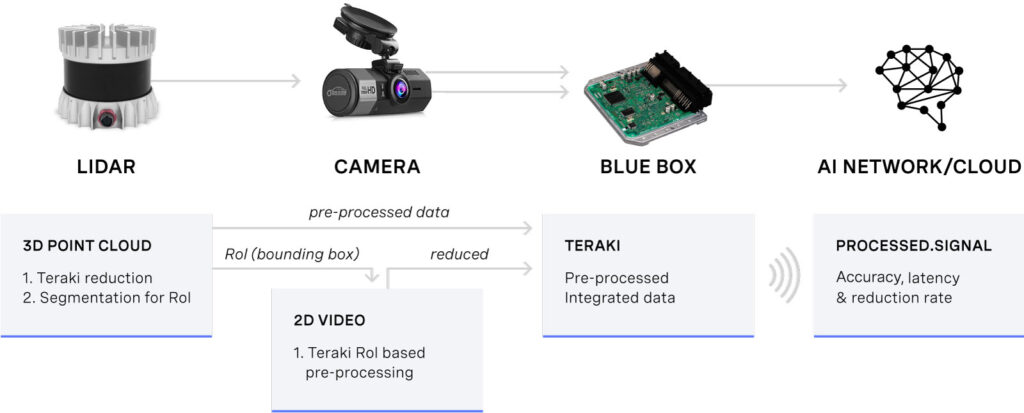
Because Ouster LiDARs collect a massive amount of data, all that data is just too heavy to be processed by the self-driving vehicles’ hardware.
The Teraki software overcomes this issue thanks to a smart detection tool. The software detects and identifies areas of interest from the Ouster 3D point cloud, compresses them and transmits only the relevant information to the computer. This data is then overlaid onto the video data.
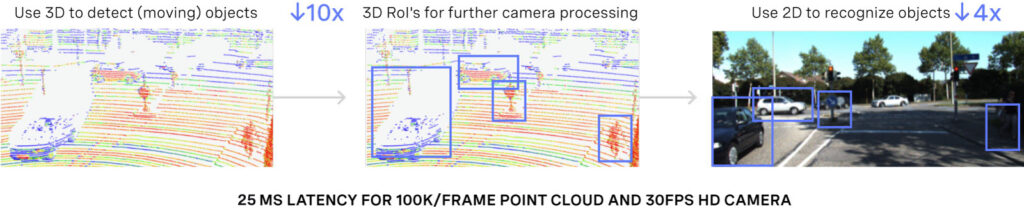
To conclude
The combination of this software with the Edge Computing technology means improved mapping, localization and perception algorithms. This results in safer vehicles that make better decisions, almost in real time. A new step towards Level 4 and 5 autonomous vehicles.
Evidence of the potential of this type of software? Robot cabs recently appeared on the streets of San Francisco!
Download our 43-pages white paper introducing the whole Ouster range
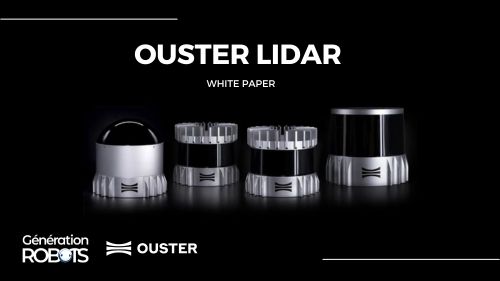