In recent years, quadruped robot performance has come on in leaps and bounds, even on difficult terrain. Mobile robots are well-suited to inspection missions, although not natively designed for tasks involving gripping. Engineers working with quadrupeds will often add external peripherals to increase the number of jobs they can perform. Which is exactly what the roboticists working for robot dog design market leader Unitree Robotics are doing. Thanks to AI and the many sensors now available on the market, a robot dog can learn how to not only pick up objects and press buttons, but also perform more complex tasks.
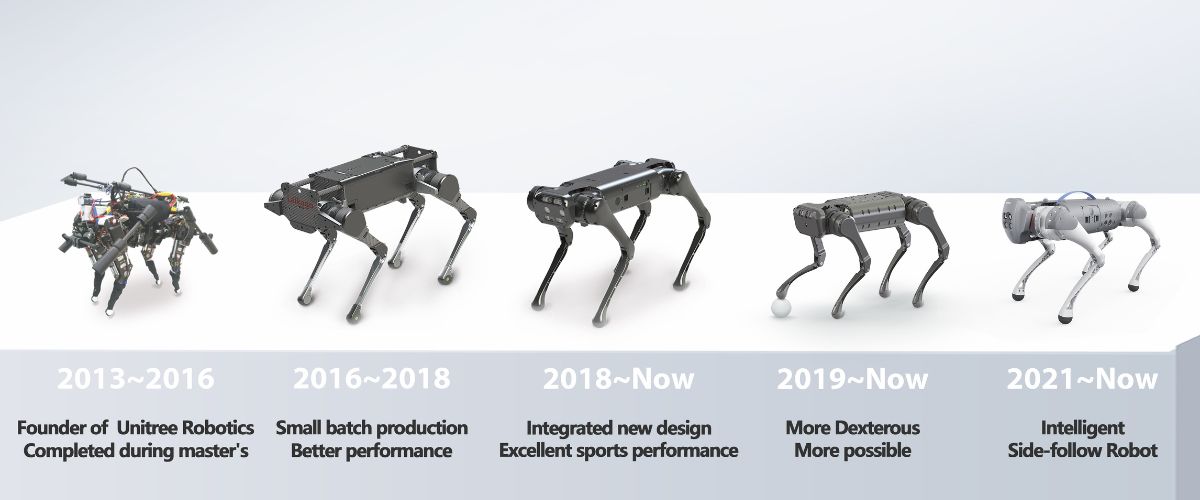
A quadruped robot equipped with an arm can be used in a much greater variety of contexts.
Traditionally, a quadruped with a robot arm will use its controller to manage walking and grasping operations separately. This is not optimal and takes a lot of time and effort to set up. There’s also a high risk of errors propagating through the different modules with the likelihood of jerky movements.
To overcome this problem, Carnegie Mellon University in Pittsburgh has turned to reinforcement learning and is testing a unified policy system to control the quadruped and the arm together. The result? Smoother, more precise movements and better arm/leg coordination.
The two students working on this project with their teacher are using the Go1 robot by Unitree Robotics, known for its cost-effective reliability and excellent performance. The arm they have chosen to mount on their robot is the WidowX 250 (6 axis) by Trossen Robotics.
Their experiment has shown how this unified policy system allows agile and dynamic behaviour in several scenarios.
The unified policy allows agile and dynamic robot body and arm behaviour
Comparison
Commercial manipulators, like the Boston Dynamics Spot Arm, represent a hefty investment for universities and research laboratories. Here, the project team came up with a cheaper alternative, using the Unitree Robotics and Trossen Robotics robots to design their own autonomous platform.
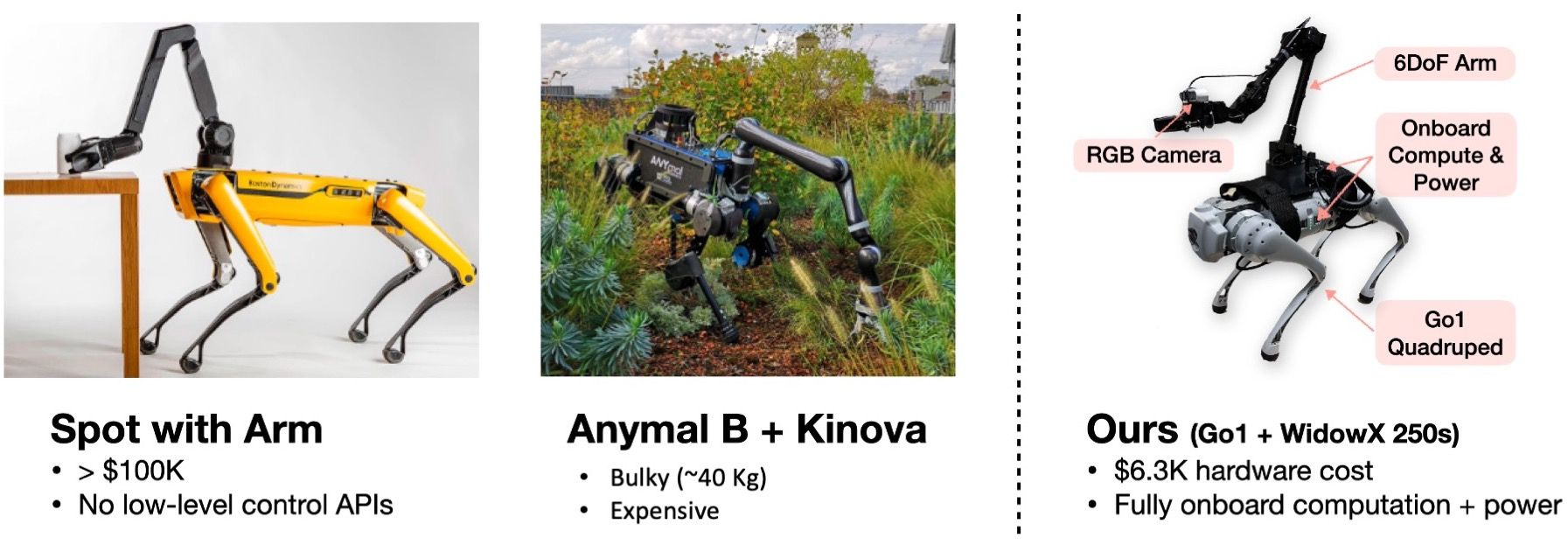
Teleoperation
The unified policy controls all joints (12 DOF for the legs, 6 DOF for the arm) in 50 Hz, using only a Raspberry Pi.
The WidowX 250s software architecture is installed on the Go1 NVIDIA Jetson using the official packages supplied by Trossen Robotics. The UDP protocol allows communication between the Raspberry Pi and the NVIDIA Jetson.
Gripper opening and closing are not supported by the unified policy, so they were controlled by a joystick during the teleoperation experiments. And the researchers used a scripted policy to manage their gripper in tests involving visual tracking.
This combination of reinforcement learning and unified policy allows fully autonomous operation of the robotics platform in 3 modes: teleoperation, visual tracking and demonstration replay. The robot can:
- place a pen in a remote cup
- pick up a cup and throw it into a high bin
- pick up an object on difficult terrain
- press a button to enter a building
Visual tracking
The robot is equipped with an Intel RealSense D435i depth camera for visual tracking.
When instructed to follow an AprilTag, the unified policy automatically adjusts the control of the robot’s whole body in order to follow it. Go1 bends its legs in coordination with the arm to ensure the camera stays near the tag.
Demonstration replay
Interested?
Our engineering design office is equipped to overcome a wide range of innovation challenges, including addition of a manipulator arm on an autonomous or semi-autonomous robot. We recently integrated collaborative arms on four omnidirectional mobile robots – you can read about it in our User Case.